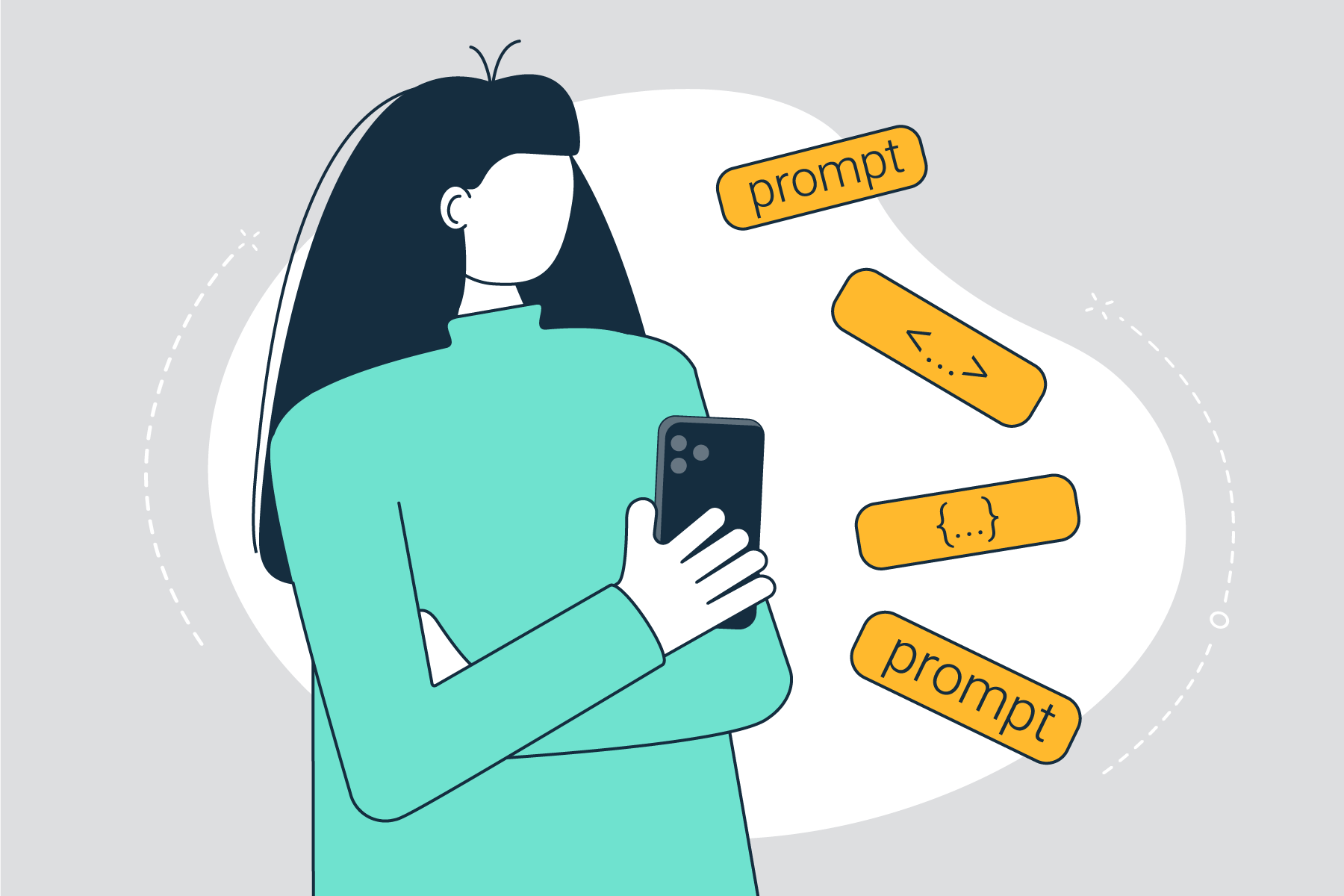
We spent years building one of the best intent-based virtual agents on the market — and then we replaced it.
When we started Espressive almost a decade ago, natural language processing (NLP) with intents and entities was considered cutting-edge. At the time, it felt like we were solving a real problem — moving away from rigid keyword search and static portals to something that actually understood what employees were asking.
And to be fair, it worked — well enough. But what was “good enough” back then doesn’t hold up today.
Over time, it became clear that intent-based virtual agents weren’t just limited — they were fundamentally flawed. Not because the implementation was bad, but because the model itself was never built to scale, adapt, or handle the complexity of real-world employee questions.
Here’s why that model was always doomed and how we moved on.
From Innovation to Obstacle
Back in the early days, using NLP to power a virtual agent felt like a breakthrough. It was a big step up from decision trees and “click here to submit a request” portals. But under the surface, it required a massive amount of configuration — defining intents, training utterances, mapping entities, and hoping it all lined up.
And it only got worse as use cases expanded. What started with a few dozen intents turned into hundreds, each with its own branching logic and maintenance overhead. What seemed scalable in theory quickly became unmanageable in practice.
The Intent Model Never Scaled
To protect our customers from that complexity, we built the Espressive Language Cloud (ELC). It centralized all the linguistic work so we could keep the bot smart without pushing that effort onto our customers. That alone was a major differentiator, especially as other vendors continued asking their customers to manage everything themselves.
Still, even with the ELC, we were fighting the limitations of the intent-based model. It could handle the obvious stuff — broad, high-volume questions — but it consistently struggled with specificity.
If an employee said, “Reset password,” no problem. But if they said something like, “I’m getting a Duo error when using my hardware key on a shared Mac,” the system would miss the mark. It wasn’t built to understand layered context or less common phrasing.
NLP was good at boulders. But the service desk gets pelted with pebbles all day long. And those pebbles — specific, nuanced, often low-volume questions — were where legacy bots broke down.
The Employee Experience Was Better—But Still Limited
Even with the best intent-based architecture available at the time — and with the ELC doing the heavy lifting — there were still limitations.
We absolutely delivered value. Employees could get answers to common questions faster, and when we didn’t have the answer, we made it easier for them to create a ticket and get help. That alone moved the needle.
But the more nuanced or specific the question, the more likely the system was to misunderstand, misroute, or fallback to generic responses. And while we captured the interaction and made escalation easier, we couldn’t always resolve the issue on the spot.
That’s where deflection rates plateaued — not because our virtual agent wasn’t trying, but because the underlying model wasn’t built to handle that level of specificity.
GenAI Changed the Game (But Most Vendors Weren't Ready)
When large language models (LLMs) arrived, they opened the door to a new kind of virtual agent — one that could understand real human language, reason through context, and take action without constant retraining.
But most vendors missed the moment.
To pick on the 800 lb. gorilla for a second, ServiceNow is a perfect example of what went wrong. Like other ITSM vendors, they approached virtual agents as a checkbox — something to bundle with their platform rather than invest in as a true solution. Their virtual agent still relied on predefined intents. GenAI only comes into play after an intent is identified. At that point, it might help rephrase a question, summarize a knowledge article, or generate a follow-up. But if the system can’t match the intent, none of that matters.
GenAI isn’t powering the understanding. It’s a cosmetic layer built on top of the same old logic.
And it wasn’t just ServiceNow. This was the pattern across the industry. Most ITSM vendors treated GenAI like a plug-in, not a foundation. And when that model couldn’t keep up — when deflection rates stagnated, and customers lost confidence — ServiceNow had to pivot. They acquired Moveworks for almost $3 billion. Not because they needed a second virtual agent, but because they didn’t have the right foundation to begin with.
Automation Was Never an Afterthought
We came from ServiceNow. But before that, we started our careers on the service desk. We’ve taken those calls. We’ve listened to the frustration. And because of that, we know exactly what employees want when they reach out for help: they want their issue resolved — not a 14-step guide on how to fix it themselves.
That’s why automation has always been core to our platform — not as an add-on, but as the foundation.
From day one, we built Espressive to prioritize outcomes. Our platform was designed to leverage the best AI available at any given time to understand the request, collect inputs, follow business logic, and resolve the issue. So, when GenAI came along, it wasn’t something we had to rethink or retrofit. It just worked because our architecture was already built to support it.
That’s the difference between bolting on intelligence and building around it. BaristaGPT, our virtual agent, doesn’t just understand what employees need but acts on it. End to end. Automatically.
And that’s what agentic really means: not just interpreting a question but owning the outcome. And we’ve been building toward that from the start.
We Didn't Retrofit. We Were Ready for This.
From the very beginning, we envisioned a future where virtual agents could understand employee language, reason through context, learn from content and systems, and take intelligent action — without needing to be trained, tuned, or scripted for every scenario.
The vision never changed. But the technology had to catch up.
When GenAI arrived, most vendors used it to enhance existing intent-based models — layering it on after an intent was identified, without fixing the core limitations. But that didn’t solve the original problem. It didn’t fix the brittle logic or the failure to understand specific, real-world employee questions. They were still stuck with boulders — and still missing the pebbles.
We took a different path. We used GenAI to eliminate the need for intents entirely. From the moment an employee starts typing to the moment their issue is resolved, GenAI is driving the entire experience. Not just for understanding, but for delivering outcomes.
This isn’t about recognizing intent. It’s about understanding the need and autonomously resolving it — whether that means walking the employee through a process, submitting a request, or providing the answer directly.
What IT Leaders Should Be Asking
By now, it’s clear that many ITSM vendors are offering virtual agents because they have to — not because they’ve actually built the architecture to support one. The result? A GenAI veneer over legacy systems that still depends on predefined intents, scripted flows, and heavy configuration.
That’s why IT leaders need to shift the conversation — from “Does it have GenAI?” to “Can it deliver value without handholding?”
Here are the questions that separate real platforms from checkbox solutions:
- What does my team need to do to ensure the system understands any topic?
- How much setup, configuration, or custom logic is required before it can provide a useful answer?
- Can it take meaningful action — or is it just providing links and summaries?
- Do we need to build and maintain this for every use case we care about?
- And how do we even identify those use cases if we don’t have a dedicated team to run the platform?
The reality is that most platforms require significant effort just to get off the ground — and even more to keep them running. If the answer to any of these questions sounds like “You'll need to build that,” then what you’re looking at isn’t a GenAI-native solution. It’s a project waiting to happen.
Some vendors have tried to reframe this challenge as innovation — introducing so-called “development studios,” as if the real breakthrough is giving you a better place to build. But the need to build is the problem in the first place.
At Espressive, we took a different path. Everything works out of the box. No builders. No prompt tuning. No intent models. And no building outcomes. You can extend if you want to — but you don’t have to. That’s the difference between a native GenAI platform and a toolkit disguised as one.
This Isn't Just a Leap. It's the Platform We Were Always Building Toward.
“Agentic” might be the word dujour — but it’s not new to us.
Our vision from the beginning was to build a platform that could understand what employees were asking, learn from the content and systems already in place, and take autonomous action — all without human configuration, tuning, or intervention.
We just needed the technology to catchup.
Today, that vision is real. BaristaGPT is a fully agentic platform — start to finish. It doesn’t rely on intent libraries or scripted flows. It doesn’t need builders, prompt engineers, or content curators. It learns from what you already have and makes real-time decisions to meet employee needs — without anyone babysitting the system.
And that, is agentic by design.
The checkbox era is over. The agentic era is already here — and we’re leading it.