
Today’s ever-changing world is pressing IT and HR leaders to review strategies and approaches to almost everything – with a focus on technologies that improve employee experience, scale organizations, improve productivity, and automate wherever possible. With this focus, AI and machine learning (ML) have become part of every professional’s vocabulary as they strive to deliver positive business outcomes. What holds organizations back, however, is having confidence that a technology investment based on AI/ML will deliver a return on investment (ROI).
The Buyer’s Journey for Leading Edge Technology
The newness, complexity, and comprehensive nature of a leading-edge technology such as AI and ML has launched many into a buyer’s journey that consists of 6 stages, beginning with awareness and ending with a decision to either buy or not buy the technology to address their business challenge. The buyer’s journey routinely intersects with B2B buying behavior clusters where buying decisions are typically categorized as:
- Independent (agreement across individuals)
- Consensus (agreement across teams/departments)
- Committee (agreement at executive/senior leadership levels)
With newer technologies, gaining consensus in any of these clusters is more challenging, particularly when it results in a significant financial investment. I’ve always leaned into a process that combines well-defined decision criteria (e.g., the what) and a documented decision process (e.g., the who and how) that provides clarity, especially when making challenging decisions.
In the end, technical and economic buyers want to put forward a selection that is:
- Aligned with business requirements
- Delivers desired positive outcomes
- Meets required timelines
- Mitigates risk
Not having a well-structured and defined evaluation process can lead to multiple negative consequences such as confusion, lack of conviction/confidence, organizational friction, and not being prepared when various questions are raised by executive management regarding any aspect of the project and process.
The Evaluation Process: Current and Future States
So how do IT and HR professionals evaluate the ROI of a technology platform, such as a conversational AI-based virtual agent like Espressive Barista? I’d like to suggest a framework that starts with documenting the current state (e.g., where the challenge/problem exists) and the future state (e.g., where the challenge/problem is reduced or eliminated).
A key elements with the current/future state framework is a preliminary ROI that documents:
- Required investment
- Quantification of benefits (hard dollar savings/improvements to mean time to resolution/ticket deflection/productivity gains)
- How it improves employee productivity, experience, and engagement
Other key elements include:
- A detailed set of required functional capabilities that the platform and project must provide to deliver the benefits reflected in the ROI.
- A well-structured proof of value (PoV) that tests a live instance of the technology being considered.
- A PoV validation event that replaces guess work with proof that the technology can perform a set of scenarios that deliver tangible benefits.
What a Well-Structured PoV Looks Like
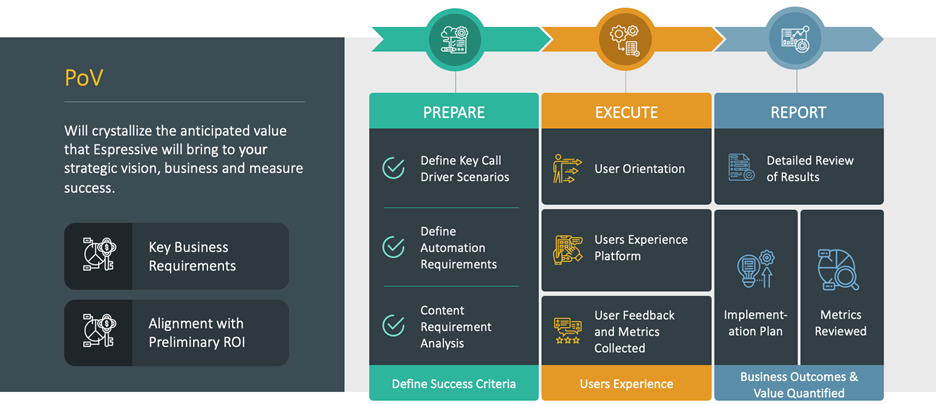
A PoV event should kick off with alignment around your strategic vision, key business objectives, and preliminary ROI calculation that quantifies the financial benefits of the solution. Then you must drive a process that starts with defining success criteria, transitions to executing use cases and user interactions, and finishes with clear reporting on the results.
The Net Result
The net result is a clear understanding of all the key elements required to make an informed decision. It is deliberate, weighs the evidence by organizing relevant information, and mitigates risk while providing clarity. In other words, you will have greater confidence that your technology investment based on AI/ML will deliver a strong return on investment and the desired positive business outcomes you envisioned.